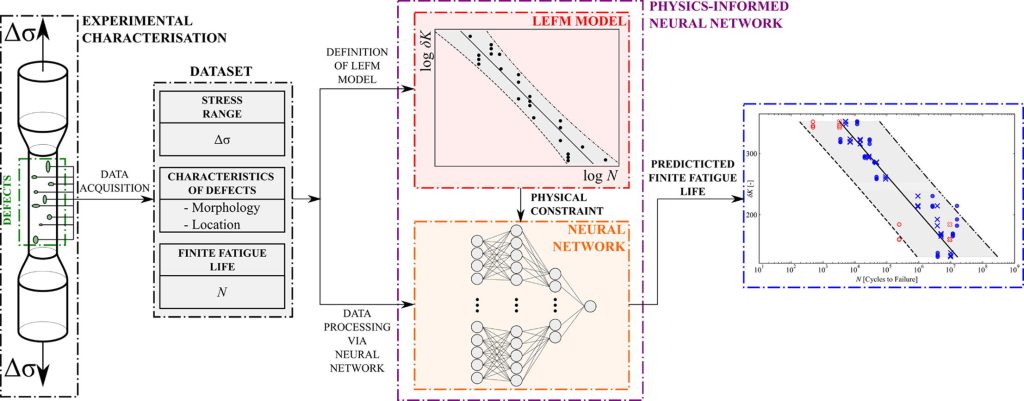
In our latest publication, we show how the accuracy of state-of-the-art fatigue predictive models, based on defects present in materials, can be significantly boosted by accounting for additional morphological features via Physics-Informed Machine Learning. We believe this is the way to go for the identification and quantification of “hidden” fatigue influencing factors while ensuring the soundness of the prediction. It’s only the beginning!
The paper published in Journal of Materials & Design (JMAD – IF 9.4) is open access.
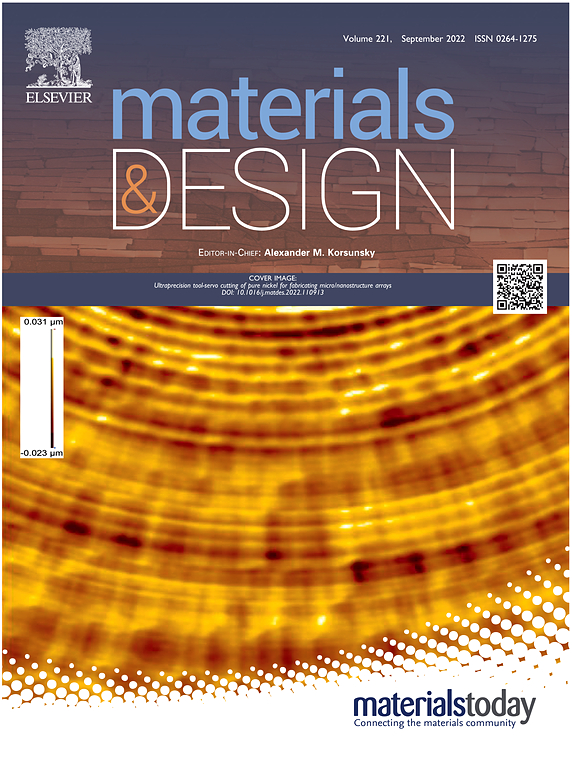
E. Salvati, A. Tognan, L. Laurenti, M. Pelegatti, F. De Bona. A Defect-Based Physics-Informed Machine Learning Framework for Fatigue Finite Life Prediction in Additive Manufacturing. (2022) Materials & Design.