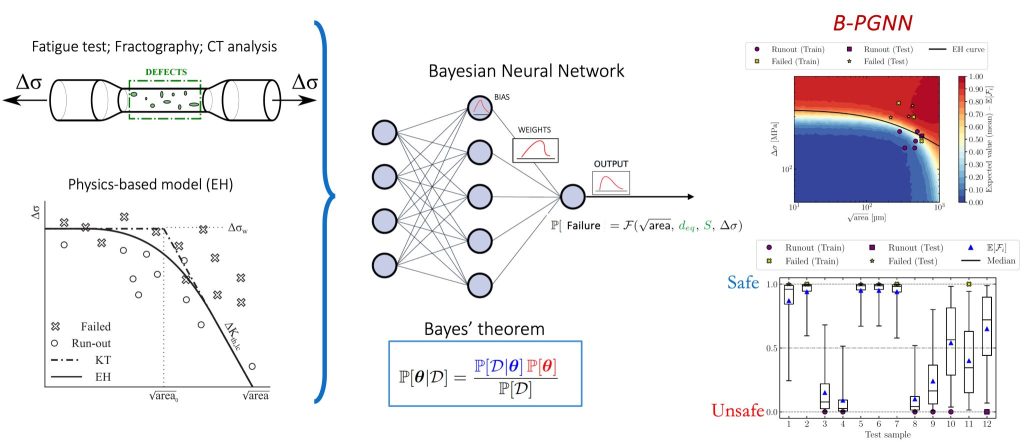
The scarcity of fatigue data makes the employment of machine learning for predictive purposes extremely challenging. On top of that, the more material characteristics and manufacturing process conditions you consider at the same time, the less these predictions appear to be robust. Following our last year’s original work adopting a Physics-Informed Neural Network (PINN) framework for fatigue finite life [1], we recently published a new Bayesian Physics-Guided Neural Network (B-PGNN) defect-based approach to tackle the issue related to the evaluation of the fatigue endurance limit in a probabilistic fashion, of great interest for design against fatigue purposes. Such a comprehensive approach fully exploits the well-consolidated El Haddad model and the strength of the Bayesian approach to overcome limitations due to small or extremely small datasets.
A great effort by Alessandro Tognan and collaborators across Europe, Luca Laurenti (TU Delft) and Andrea Patanè (Trinity College Dublin) for their great effort!
The paper has been published this month on Computer Methods in Applied Mechanics and Engineering (IF 7.2) and is free to read to anyone interested at:
ReserchGate:
https://lnkd.in/ekgZ3GBx
Science Direct
https://lnkd.in/eVzqwJmm
Further readings:
[1] E. Salvati, A. Tognan, L. Laurenti, M. Pelegatti, F. De Bona. A Defect-Based Physics-Informed Machine Learning Framework for Fatigue Finite Life Prediction in Additive Manufacturing. (2022) Materials & Design. DOI: 10.1016/j.matdes.2022.111089
[2] E. Avoledo, A. Tognan, E. Salvati. Quantification of Uncertainty in a Defect-based Physics-Informed Neural Network for Fatigue Evaluation and Insights on Influencing Factors. (2023) Engineering Fracture Mechanics. DOI: 10.1016/j.engfracmech.2023.109595
[3] A. Tognan, E. Salvati. Probabilistic Defect-based Modelling of Fatigue Strength for Incomplete Datasets Assisted by Literature Data. (2023) International Journal of Fatigue. DOI: 10.1016/j.ijfatigue.2023.107665
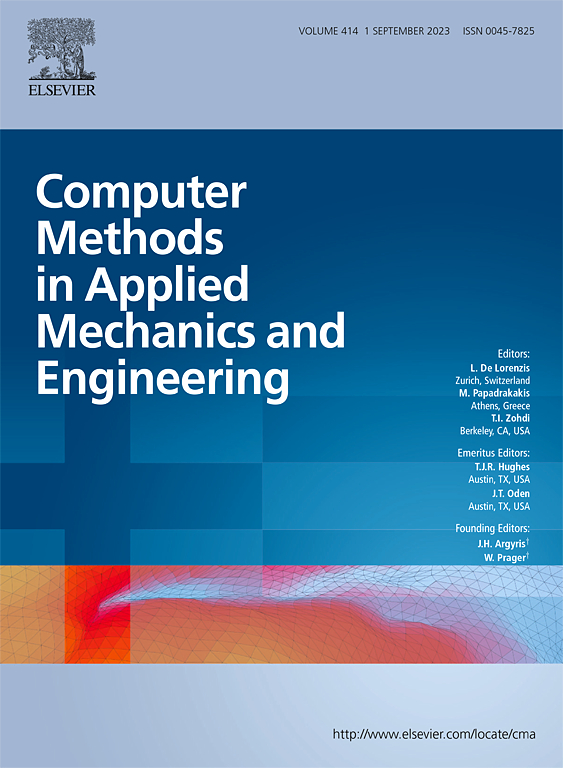
A. Tognan, A. Patanè, L, Laurenti, E. Salvati. A Bayesian Defect-based Physics-guided Neural Network Model for Probabilistic Fatigue Endurance Limit Evaluation. (2024) Computer Methods in Applied Mechanics and Engineering: DOI:10.1016/j.cma.2023.116521