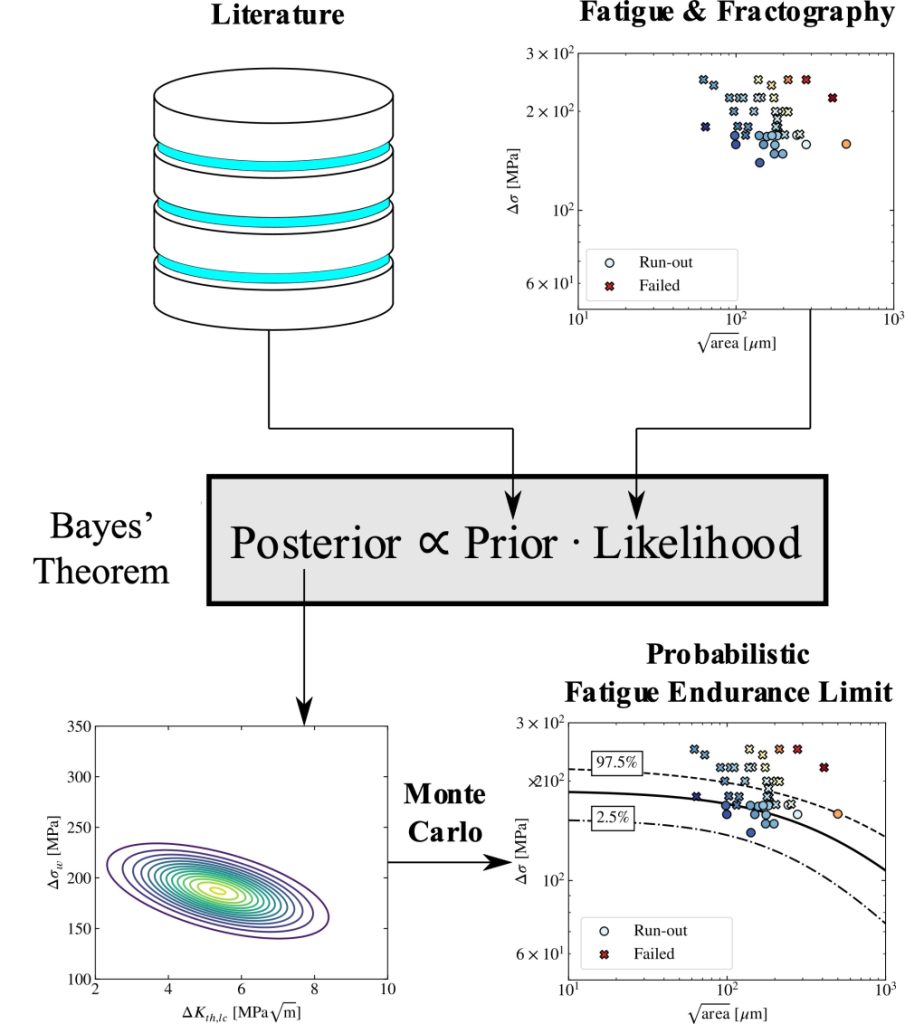
The experimental evaluation of the fatigue endurance limit for a specific material is often very demanding, especially when dealing with materials containing defects, such as pores or cracks. On the other hand, the exploitation of existing results from the literature is very problematic due to the material’s susceptibility to their manufacturing route, other than its chemical composition: often even similar processes lead to remarkably different fatigue behaviours. Is it possible to draw a reliable fatigue assessment regardless of the scarcity of the experimental data?
Our latest publication showcases a novel Machine Learning Bayesian framework to assess the fatigue endurance limit of metallic alloys when an insufficient number of fatigue tests are available. To do so, information from literature resources is gathered and cast within this framework to assess the sought material characteristic in a probabilistic manner.
The developed computational framework will represent a handy probabilistic tool to assess the fatigue endurance limit in numerous engineering scenarios involving defective materials when limited fatigue tests are obtainable – an essential requirement for a reliable design against fatigue failure.
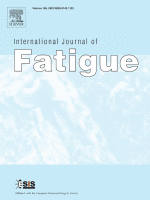
A. Tognan, E. Salvati. Probabilistic Defect-based Modelling of Fatigue Strength for Incomplete Datasets Assisted by Literature Data. (2023) International Journal of Fatigue. DOI: 10.1016/j.ijfatigue.2023.107665